

Using PLA Wood, from Hatchbox.

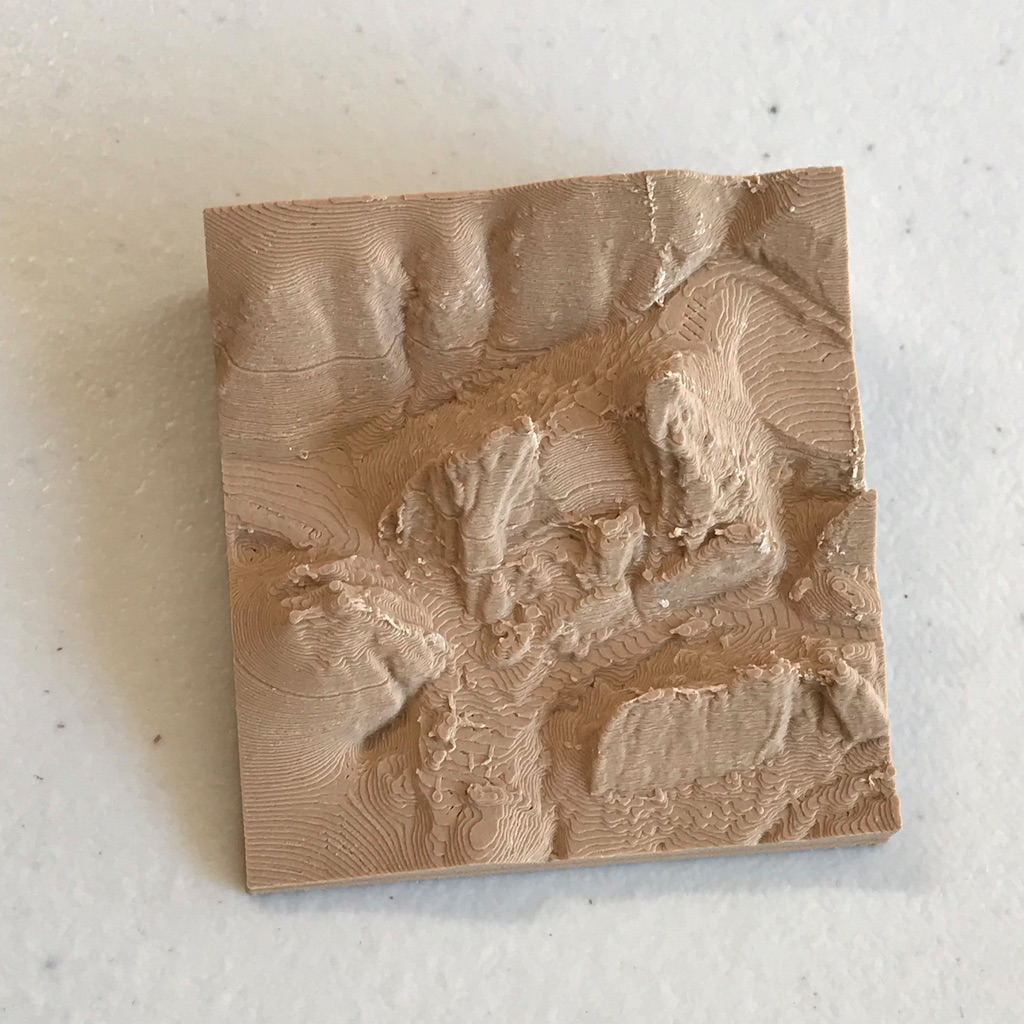
Using PLA Wood, from Hatchbox.
Meshlab can do this, if you don’t have access to a linux machine and use
ctmconv red-rocks-smrf-only-delaunay.ply red-rocks-smrf-only-delaunay.ply
Meshlab seems great, may even be as useful as Blender.
On Windows 10, the default viewer.
I’m going to use the Creality Slicer (which is based off Cura). It doesn’t quite fit.
This seems reasonable to print.
Will fire up the printer and see!
Using Blender, I add a cube and played around with the positioning. I found the Blender UI to be very non-intuitive.
Then export as PLY file. It looks like this.
I was playing around with the lidar dataset from UCSD and visualizing it on Entwine.
Wanted to share some beautiful cool pictures. Lidar is very interesting. Here are the links to play this yourself.
https://s3-us-west-2.amazonaws.com/usgs-lidar-public/ARRA-CA_GoldenGate_2010/ept.json
Looking at the data format, it the bounding boxes for the octo-tree here are:
Points
-13632855.894, 4553466.772, 1.042
-13635492.356, 4553196.558, 1.418
-13634390.511, 4555612.511, 72.595
-13633919.870, 4551994.285, 81.365
Ones in bold are the min/max vals, thus forming our X, Y bounds.
{
# type: readers.ept
bounds: ([-13635492.356,-13632855.894], [4555612.511, 4551994.285])
filename: https://s3-us-west-2.amazonaws.com/usgs-lidar-public/ARRA-CA_GoldenGate_2010/ept.json
resolution: 0.5
# up to 0.5m resolutions
}
Actually this didn’t return any data. So I’m doing another selection with larger area.
The pipeline
{
"pipeline": [
{
"bounds": "([-13637879,-13630062], [4549481,4557084])",
"filename": "https://s3-us-west-2.amazonaws.com/usgs-lidar-public/ARRA-CA_GoldenGate_2010/ept.json",
"type": "readers.ept"
},
{
"filename": "golden-gate.laz",
"type": "writers.las"
}
]
}
474M May 4 23:50 golden-gate.laz
I put together and loaded this data, visualized with potree:
That’s it folks!
Looking at Red Rocks.
# This is a hjson file, https://hjson.org/
# Linux bash
#GET=https://github.com/hjson/hjson-go/releases/download/v3.0.0/linux_amd64.tar.gz
# macOS bash
#GET=https://github.com/hjson/hjson-go/releases/download/v3.0.0/darwin_amd64.tar.gz
# Install
#curl -sSL $GET | sudo tar -xz -C /usr/local/bin
# Translate to Json
#hjson -j pipeline.hjson > pipeline.json
#pdal pipeline pipeline.json --verbose 8
{
pipeline:
[
# Input
{
# read from our ept server
# up to 0.5m resolutions
# type: readers.ept
# bounds: ([802000, 802500], [2493000, 2493500])
# filename: http://localhost:8080/ept.json
filename: red-rocks.laz
# filename: http://na.entwine.io/red-rocks/ept.json
# resolution: 0.5
}
# {
# # read from our las file
# type: readers.las
# filename: small500-no-outliers.laz
# }
# Filters
{
# adds a classification value of 7 to the noise points
type: filters.outlier
# method: radius
# radius: 1.0
# min_k: 8 # min number of neighbors in radius
method: statistical
mean_k: 8
multiplier: 3
}
{
# voxel-based sampling filter
# reduce the size of the pc
# cell size of 0.2 meters in xyz
type: filters.voxelcenternearestneighbor
cell: 0.1
}
{
# Need to assign point cloud dimension NumberOfReturns 1
# Otherwise: "No returns to process."
type:filters.assign
assignment : NumberOfReturns[0:0]=1
}
{
# Ground classification, ignore the noise points
type: filters.smrf
ignore:Classification[7:7]
}
{
# only allow ground classified points
type: filters.range
limits: Classification[2:2]
}
{
# OPTIONAL
# turn this into a DEM 3D model
# do not use multiple types
# type: filters.delaunay
type: filters.poisson
}
# Output
# # OPTIONAL PLY IF DEM
{
# write to ply
type:writers.ply
filename: red-rocks-smrf-only-poisson.ply
faces:true
storage_mode: default
}
# Output
# {
# # write to laz
# type:writers.las
# filename: red-rocks-ground.laz
# }
]
}
https://gist.github.com/sunapi386/9a9ece302d646ee80a72fc494423a633
To go a little more “detailed”, I put depth to 12. The file went from 84M to 789M. And it is definitely overkill for 3D printing.
Program failed to compute the grid projection.
pdal pipeline dtm-gdal.json --verbose 8
(PDAL Debug) Debugging...
(pdal pipeline Debug) Attempting to load plugin '/usr/local/lib/libpdal_plugin_filter_gridprojection.so'.
(pdal pipeline Debug) Loaded plugin '/usr/local/lib/libpdal_plugin_filter_gridprojection.so'.
(pdal pipeline Debug) Initialized plugin '/usr/local/lib/libpdal_plugin_filter_gridprojection.so'.
(pdal pipeline readers.las Debug) GDAL debug: OGRSpatialReference::Validate: No root pointer.
(pdal pipeline readers.las Debug) GDAL debug: OGRSpatialReference::Validate: No root pointer.
(pdal pipeline readers.las Debug) GDAL debug: OGRSpatialReference::Validate: No root pointer.
(pdal pipeline Debug) Executing pipeline in standard mode.
(pdal pipeline filters.gridprojection Debug) Process GridProjectionFilter...
[pcl::GridProjection::getBoundingBox] Size of Bounding Box is [5.500000, 6.000000, 5.000000]
[pcl::GridProjection::getBoundingBox] Lower left point is [-2.500000, -2.500000, -2.500000]
[pcl::GridProjection::getBoundingBox] Upper left point is [3.000000, 3.500000, 2.500000]
[pcl::GridProjection::getBoundingBox] Padding size: 3
[pcl::GridProjection::getBoundingBox] Leaf size: 0.500000
(pdal pipeline filters.gridprojection Debug) 3141373 before, 180 after
(pdal pipeline filters.gridprojection Debug) 180
double free or corruption (!prev)
fish: “pdal pipeline dtm-gdal.json --v…” terminated by signal SIGABRT (Abort)
Cura can take STL inputs. Converting the PLY into STL is simple.
sudo apt install openctm-tools
Then ctmconv red-rocks-smrf-only-delaunay.ply red-rocks-smrf-only-delaunay.stl
can convert ply to stl
ctmviewer red-rocks-smrf-only-delaunay.ply
visualizes the ply. Which is what I used above.
Looks like the Poisson is prettier.
I’ll continue writing this latter, until I have something printed. 🙂